April 14, 2020
PhD Collaboration with the Vrije Universiteit Amsterdam
The Datalab is known to make innovative and advanced solutions available for Pon companies. Many solutions are pushing the boundaries of currently available software by using advanced and recently developed algorithms. To keep the knowledge of the Datalab on the highest possible level, we started a PhD collaboration with the Vrije Universiteit in September 2018. By combining the theoretical knowledge of the applied mathematics and computer science departments with the practical challenges of Pon companies, we believe we can keep pushing the boundaries of our algorithms and hereby make Pon smarter every day.
The collaboration with the VU is specifically oriented towards PhD research. At the moment, two members of the Datalab are working towards achieving their PhD whilst working on practical problems of Pon companies. They work from the university one day per week, the others are spent collaborating with the team and solving various problems. In September 2018, Jesper started the first traject, followed by Robin in October 2019.
Jesper
Jesper is planning to do four major projects in four years. The first project has recently been completed at Shuttel, with the main question being: Can we predict mobility choices of individuals? Shuttel provides various types of mobility to its customers and wants to be as relevant as possible. Therefore, knowing their needs is crucial. Predicting mobility choices is difficult, as it depends on a large amount of factors. By taking into account travel distance, time, congestion, weather, infrastructure, repeating trips, different start- and end locations, and aggregated personal statistics we can predict with 97% certainty which transport type will be chosen for a certain trip. Currently, we are working on implementing the algorithm in order to make Shuttel even more relevant for its customers.
The second project is currently on-going at Gazelle, concerning integrating Point of Sale (PoS) data in Gazelle’s reporting, website, and supply chain. This data source concerns the daily sales and stock levels of Gazelle’s dealers, thereby having huge potential for Gazelle’s decision making. However, the raw data needs to be cleaned before it can be interpreted and this interpretation can be misleading. In a few months from now, we can not only create accurate forecasts on the historical sales, however, additionally create an accurate estimation of the effort it took to sell this bike. Hereby we can further optimize and improve the supply chain of Gazelle and help them be more relevant to their customers as well.
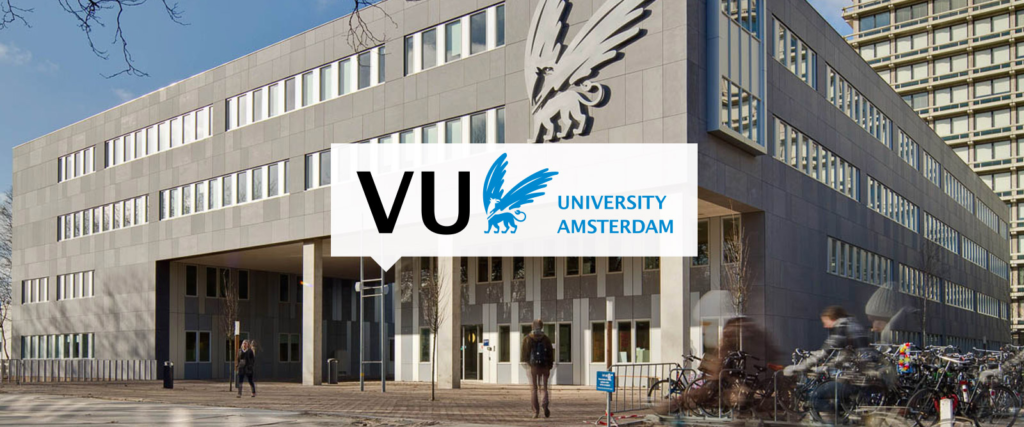
Robin
Robin is focusing on computer vision and predictive modelling for the mobility sector. In his first project, he answered the question: can we locate and classify vehicle damages from pictures using artificial intelligence? During this research, we worked together with Pon Logistics, where more than 120,000 vehicles per year receive a manual damage inspection. This process is currently time consuming, resulting in a bottleneck at the inbound process. To address this, we developed a self learning model which is able to predict the damage location and type from high resolution images. By providing thousands of images with damaged vehicles, the model is able to learn the general characteristics of a damage. Using a large diversity in vehicle brand and model, a general system is developed which can detect damages on vehicles it has never seen before. Automatic vehicle damage detection is not only relevant for Pon Logistics but has a much wider application, where it can be used for car sharing, rental, and delivery companies. Although the focus is mainly on vehicles, we are already investigating if we can extend this research to other mobility/products.
Many companies within Pon are using forecasting to predict future sales and to adapt their stock levels on it. A traditional way of forecasting is to look at each item and create a forecast based on the historical sales. This second research aims to improve the forecasting per item by incorporating the sales patterns of similar items. It is already common practice to include the historical sales of different item colour, item configurations, or item groups when forecasting one item. However, this does not look at items that are totally different but behave similar in terms of historical sales. Those items are frequently in different product groups. Therefore, this research looks at sales relationships between items and uses this to improve the sales forecasting for each individual item. Using this, a major improvement in the forecasting accuracy is achieved for items which are sold infrequently and are difficult to forecast with normal methods. Hereby, we can further improve the forecasting applications provided by the Pon Datalab.
Robin van Ruitenbeek
AI SpecialistJesper Slik
Senior AI Specialist