October 24, 2019
PEPP AI Challenge – Excavator Image Recognition
This year, the Digital Innovation Lab organized the Pon Equipment and Pon Power AI challenge. This challenge was focused around Artificial Intelligence initiatives that could prove valuable for the business of PEPP. The Datalab participated using an image recognition algoritme to recognize excavators and other equipment on the websites of potential customers of Pon Compact. As the name suggests, Pon Compact’s business is centered around (relatively) small Caterpillar equipment. The last few years Pon Compact grew in market share, but their ambition is to grow further and become one of the market leaders in the compact segment.
To further increase market share, Pon Compact needs to find high quality leads and turn these into new customers. The Datalab already worked with Pon Compact on previous Lead Generation programs. In these programs we classify leads based on the information on the website. For instance, if a company mentions it is mainly active in the earth moving market, this indicates that it may be an interesting company for Pon Compact.
The last Lead Generation program has seen a conversion rate of roughly 30%. That already sounds like a high percentage, but on the downside means that you spend 70% of your resources on leads that do not convert. To improve the conversion rate, we decided to not only look at the text on a company website, but also analyze the photos with image recognition. Image recognition is a technique which can be used to identify objects on images. Image recognition will give Lead Generation a new dimension as you will automatically be able to tell what sort of photos a company publishes.
To recognize excavators in photos we had to teach an algorithm what an excavator looks like. Furthermore, we also had to make sure it could distinguish between small machines relevant to Pon Compact (BCP) and the bigger machines (GCI). We collected over 90K images of both types of machines and fed these to the model to train it in the recognition. The resulting model could accurately recognize excavators and more importantly, distinguish between machine size.
We tested the model on 84 verified leads of which we knew whether or not they possessed the relevant machines. Of the 27 leads that had no excavator, we found that 74% had no images with an excavator on their homepage. On the other hand, of the 57 leads that had an excavator we determined that 30% of them had images with an excavator on the home page. This shows there are still some false positives and negatives, but it also shows that the conversion rate could be increased analyzing images. Moreover, due to the limited scope of this experiment we did not look further than the images on the homepage. As a next step we plan to include more images from other pages on the websites.
For more information, please read this article.
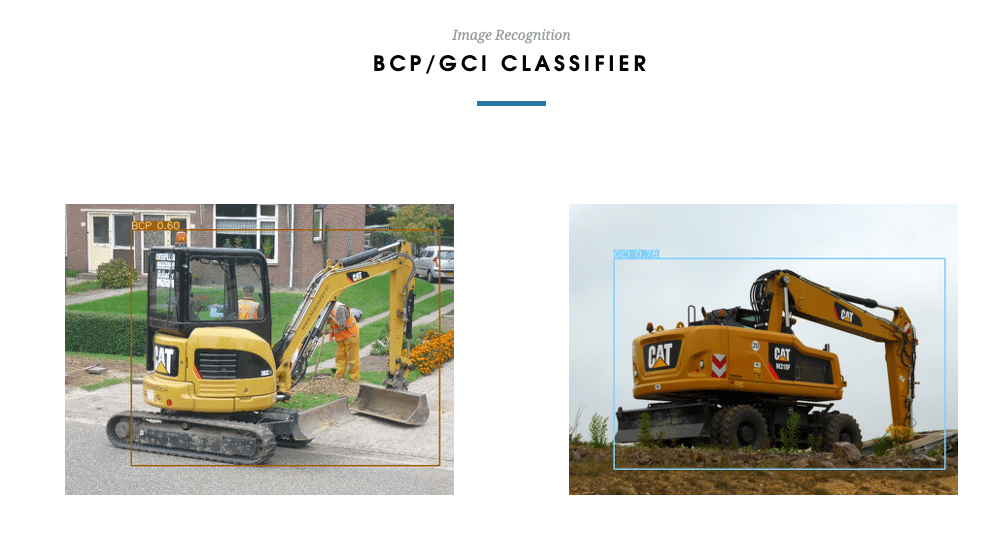
Kevin Haver
Lead Data ScientistKoen Haenen
Lead Data ScientistKasper Meijjer
Senior Data ScientistRobin van Ruitenbeek
AI SpecialistKirsten Kniep
Data Scientist